ORIGINAL ARTICLE
CHAGAS, Edgar Thiago De Oliveira [1]
CHAGAS, Edgar Thiago De Oliveira. Deep Learning and its applications today. Revista Científica Multidisciplinar Núcleo do Conhecimento. 04 year, Ed. 05, Vol. 04, pp. 05-26 may 2019. ISSN: 2448-0959
SUMMARY
Artificial intelligence is no longer a plot for fiction movies. Research in this field increases every day and provides new insights into Machine learning. Deep learning methods, also known as Deep Learning, are currently used on many fronts such as facial recognition in social networks, automated cars and even some diagnoses in the field of medicine. Deep Learning allows computational models composed of countless processing layers to “learn” representations of data with varying levels of abstraction. These methods improved speech recognition, visual objects, object detection, among possibilities. However, this technology is still poorly known, and the purpose of this study is to clarify how Deep Learning works and to demonstrate its current applications. Of course, with the dissemination of this knowledge, deep learning can, in the near future, present other applications, even more important for all mankind.
Keywords: Deep Learning, machine learning, IA, machinery Learning, intelligence.
INTRODUCTION
Deep learning is understood as a branch of machine Learning, which is based on a group of algorithms that seek to shape high-level abstractions of data using a deep graph with several layers of processing, Composed of several linear and nonlinear alterations.
Deep Learning works the computer system to perform tasks such as speech recognition, image identification and projection. Rather than organizing the information to act through predetermined equations, this learning determines basic patterns of this information and teaches computers to develop through the identification of patterns in processing layers.
This kind of learning is a comprehensive branch of Machine Learning methods based on learning representations of information. In this sense, Deep Learning is a set of learning machine algorithms that try to integrate multiple levels, which are recognized statistical models that correspond to varying levels of definitions. Lower levels help define many notions of higher levels.
There are countless current researches in this area of artificial intelligence. The improvement in Deep Learning techniques has implemented improvements in the ability of computers to understand what is requested. Research in this field seeks to promote better representations and elaborate models to identify these representations from information not labeled on a large scale, some as a basis in the findings of neuroscience and in the interpretation of Data processing and communication patterns in the nervous system. Since 2006, this kind of learning has emerged as a new branch of machine learning research[2].
Recently, new techniques have been developed from Deep Learning, which have impacted several studies on signal processing and pattern identification. Note a range of new problematic commands that can be solved through these techniques, including Machine Learning and artificial intelligence key points.
There is a great deal of media attention, according to Yan[3]g et al, on the advances achieved in this area. Large technology organizations have applied many investments in Deep Learning research and their new applications.
Deep Learning encompasses learning at various levels of representation and intangibility that help in the process of understanding information, images, sounds and texts.
Among the exhibitions available on deep learning, it is possible to identify two striking points. The first demonstrates that they are models formed by countless layers or steps of non-linear data processing and are also supervised learning practices or not, of the representation of attributions in later and intangible layers.
It is understood that Deep Learning is in the joints between the branches of neural network research, AI, graphic modeling, identification and optimization of patterns and signal processing. The attention given to deep learning is due to the improvement of the chip processing skill, the considerable increase in the size of the information used for training and the recent advances in the studies in Machine Learning and processing of signals.
This progress allowed Deep Learning practices to effectively exploit complex and non-linear applications, identify representations of distributed and hierarchical resources, and enable the effective use of Labeled and unlabeled information.[4]
Deep Learning refers to a comprehensive class of Machine Learning methods and projects, which bring together the characteristic of using many layers of non-linear processed data of a hierarchical nature. Due to the use of these methods and projects, a large part of the studies in this sphere can be classified into three main sets, according to Pa[5]ng et al, which are the deep networks for unsupervised learning; Supervised and hybrid.
Deep networks for unsupervised learning are available to apprehend the high sequence correlation of the analyzed or identifiable information for verification or association of standards when there is no data on the stereotypes of the classes Available in the database. Learning of attribution or unsupervised representation refers to deep networks. Also, you can search for the assignment of grouped statistical distributions of the visible data and its related classes when available, and may be covered as part of the visible data.
Deep neural networks for supervised learning should provide discrimination to classify patterns, usually individualizing the subsequent distributions of classes linked to the visible information, which are always Available for this supervised learning, also referred to as deep discriminative networks.
The deep hybrid networks are highlighted by the discrimination identified with the results of generative or unsupervised deep networks, which can be achieved through the improvement and/or regularization of the deep supervised networks. Its attributions can also be achieved when the discriminatory guidelines for supervised learning are used to assess standards in any generative or unsupervised deep network[6].
The deep and recurrent networks are models that present a high performance in issues of identification of questionable patterns in Ivain and speech[7]. Despite its power of representation, the great difficulty in shaping deep neural networks with generic use persists to the present day. In relation to the recurrent neural networks, studies by Hinton e[8]t al initiated the molding in layers.
The present study aims to clarify the progress of Deep Learning and its applications according to the most recent researches. To do this, a qualitative descriptive research will be conducted, with the use of books, theses, articles and websites to conceptually the advances in the area of artificial intelligence and especially in deep learning.
There has been a growing interest in Machine Learning since the last decade, given that there is an ever-increasing interaction between applications, whether mobile or computer devices, with individuals, through programs for detecting spam, Recognition in photos on social networks, smartphones with facial recognition, among other applications. According to Gartne[9]r all corporate programs will have some function linked to Machine Learning up to the year 2020. These elements seek to justify the elaboration of this study.
HISTORICAL DEVELOPMENT OF DEEP LEARNING
Artificial intelligence is not a recent discovery. It comes from the decade of 1950, but despite the evolution of its structure, some aspects of credibility were lacking. One such aspect is the volume of data, originated in wide variety and speed, enabling the creation of standards with high levels of accuracy. However, a relevant point was on how large Machine Learning models were processed with large amounts of information, because computers could not perform such action.
At that moment, the second aspect referred to parallel programming in GPUs was identified. The graphic processing units, which enable the realization of mathematical operations in parallel, especially those with matrices and vectors, which are present in models of artificial networks, allowed the current evolution, i.e. the Big Data Summation (large data volume); Parallel processing and Machine Learning models present as a result artificial intelligence.
The basic unit of an artificial neural network is a mathematical neuron, also called a node, based on the biological neuron. The links between these mathematical neurons are related to those of biological brains, and especially in the way these associations develop over time, called “training”.
Between the second half of the decade of 80 and the beginning of the decade of 90, several relevant advances in the structure of artificial networks happened. However, the amount of time and information needed to achieve good results procrastinated the adoption, affecting the interest on artificial intelligence.
In the early 2000 years, the power of computing expanded and the market experienced a “boom” of computational techniques that were not possible before. It was when Deep Learning emerged from the great computational growth of that time as an essential mechanism for the elaboration of Artificial intelligence systems, winning several Machine Learning competitions. The interest in deep learning continues to grow until the present day and several commercial solutions emerge at all times.
Over time, several researches were created in order to simulate brain functioning, especially during the learning process to create intelligent systems that could recreate tasks such as classification and pattern recognition, among Other activities. The conclusions of these studies generated the model of artificial neuron, placed later in an interconnected network called neural network.
In 1943, Warren McCulloch, neurophysiologist, and Walter Pitts, mathematician, created a simple neural network using electrical circuits and elaborated a computer model for neural networks based on mathematical concepts and algorithms called threshold Logic or threshold logic, which allowed research on the neural network divided into two strands: focusing on the biological process of the brain and another focusing on the application of these neural networks aimed at artificial intelligence.[10]
Donald Heb[11]b, in 1949, wrote a work where he reported that neural circuits are strengthened the more they are used, as the essence of learning. With the advancement of computers in the 1950 decade, the idea of a neural network gained strength and Natha[12]nial Rochester from IBM’s study labs tried to constitute one, yet did not succeed.
Dartmouth’s summer research project on[13] Artificial Intelligence, in 1956, boosted neural networks, as well as artificial intelligence, encouraging research in this area in relation to neural processing. In the years that followed, John Von Neumann imitated simple functions of neurons with vacuum tubes or telegraphs, while Frank Rosenblatt initiated the Perceptron project, analyzing the functioning of the eye of a fly. The result of this research was a hardware, which is the oldest neural network used up to the present day. However, the Perceptron is very limited, which was proven by Marvin and Papert[14]
Figure 1: Neural network structure
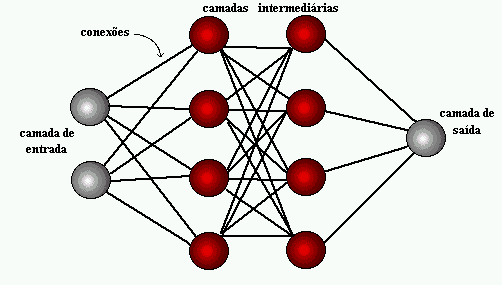
A few years later, in 1959, Bernard Widrow and Marcian Hoff developed two models called “Adaline” and “Madaline”. The nomenclature derives from the use of multiple elements: ADAptive LINear. Adaline was created to identify binary patterns in order to make predictions about next bit, while “Madaline” was the first neural network applied to a real problem, using an adaptive filter. The system is still in use, but only commercial.[15]
The progress achieved previously led to the belief that the potential of neural networks was limited to electronics. It was questioned about the impact that “intelligent machines” would have on man and society as a whole.
The debate on how Artificial intelligence would affect man, raised criticism about research in neural networks which caused a reduction in funding and, consequently, studies in the area, which remained until 1981.
The following year, several events reacthe interest in this field. John Hopfield of Caltech presented an approach to creating useful devices, demonstrating his assignments[16]. In 1985, the American Institute of Physics began an annual meeting called Neural Networks for computation. In 1986, the media began to report the neural networks of several layers, and three researchers presented similar ideas, called Backpropagation networks, because they distribute patterns identification failures across the network.
The hybrid networks had only two layers, while the Backpropagation netwo[17]rks present many, so that this network retains information slower, because they need thousands of iterations to learn, but also present more results Accurate. Already in 1987, there was the first international conference on Neural Networks of the Institute of Electrical and Electronic Engineer’s (IEEE).
In the year 1989, scientists created algorithms that used deep neural networks, but the time of ‘ learning ‘ was very long, which prevented its application to reality. In 1992, Juyang Weng Diulga The Cresceptron method to perform the recognition of 3D objects from tumultuous scenes.
In the mid-2000 years, the term Deep Learning or deep learning begins to be diffused after an article by Geoffrey Hinton and Ruslan Salakhut[18]dinov, which demonstrated how a multi-layered neural network could be previously trained, one layer at a time .
In 2009, the Neural Network systems processing Workshop on Deep Learning for voice recognition takes place and it is verified that with an extensive data group, neural networks do not need prior training and failure rates fall Significantly[19].
In 2012, researches provided identification algorithms of artificial patterns with human performance in some tasks. And the Google algorithm identifies felines.
In 2015, Facebook uses Deep Learning to automatically mark and recognize users in photos. The algorithms perform facial recognition tasks using deep networks. In the year 2017, there was a large-scale adoption of deep learning in various business applications and mobile devices, as well as progress in research[20].
Deep Learning’s commitment is to demonstrate that a fairly extensive set of data, fast processors and a fairly sophisticated algorithm, makes it possible for computers to perform tasks such as recognizing images and voice, among other Possibilities.
The research on neural networks has gained prominence with promising attributions presented by the neural network models created, due to the recent technological innovations of implementation that allow to develop audacious neural structures Parallel to hardware, achieving satisfactory performances of these systems, with superior performance to conventional systems, including. The evolution of neural networks is Deep Learning.
DEEP LEARNING
It is initially up to differentiate artificial intelligence, Machine Learning and Deep Learning.
Figure 2: Artificial Intelligence, Machine Learning and Deep Learning
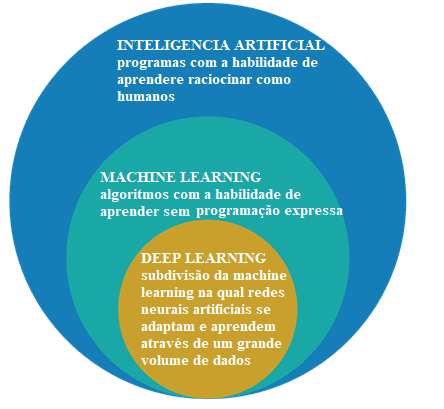
The field of study of artificial intelligence is the research and design of intelligent sources, that is, a system that can make decisions based on a characteristic considered intelligent. In artificial intelligence there are several methods that model this characteristic and among them is the sphere of Machine Learning, where decisions are made (intelligence) based on examples and not a determined programming.
Machine Learning algorithms require information to remove features and learings that can be used to make future decisions. Deep Learning is a subgroup of Machine Learning techniques, which generally use deep neural networks and need a large amount of information for training[21].
According to Santana[22] there are some differences between the techniques of Machine Learning and the methods of Deep Learning, and the main ones are the need and the impact of data volume, computational power and flexibility in the modeling of problems.
Machine Learning needs data to identify patterns, but there are two issues regarding the data that refers to the dimensionality and stagnation of performance by introducing more data beyond the behaved limit. It is verified that there is a reduction in significant performance when this occurs. In relation to dimensionality the same occurs, as there are many information to detect, through the classical techniques the dimension of the problem.
Figure 3: Comparison of deep learning with other algorithms regarding the amount of data.
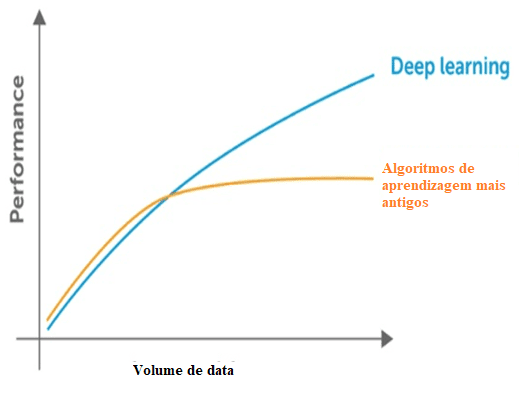
The classical techniques also present a saturation point in relation to the amount of data, that is, have a maximum limit to extract the information, which does not occur with deep learning, created to work with a large volume of data.
In relation to the computational power for deep learning, its structures are complex and require a large volume of data for its training, which demonstrates its dependence on a large computational power to implement these practices. While other classic practices need a lot of computational power as a CPU, Deep Learning techniques are superior.
Searches related to parallel computing and the use of GPUs with CUDA-Compute Unified Device Architecture or Unified computing device architecture have initiated Deep Learning, as it was unfeasible with the use of a simple CPU.
In a comparison with the training of a deep Neural network or deep learning with the utilization of a CPU, it turns out that it would be impossible to obtain satisfactory results even with prolonged training.
Deep Learning, also known as deep learning, is a part of machine Learning, and it applies algorithms to process data and reproduce the processing performed by the human brain.
Deep learning uses layers of mathematical neurons to process data, identify speech, and recognize objects. The data is transmitted through each layer, with the output from the previous layer granting input to the next layer. The first layer in a network is called the input layer and the last is the output layer. The intermediate layers are called hidden layers, and each layer of the network is formed by a simple and uniform algorithm that encompasses a kind of activation function.
Figure 4: Simple Neural Network and deep Neural network or Deep Learning
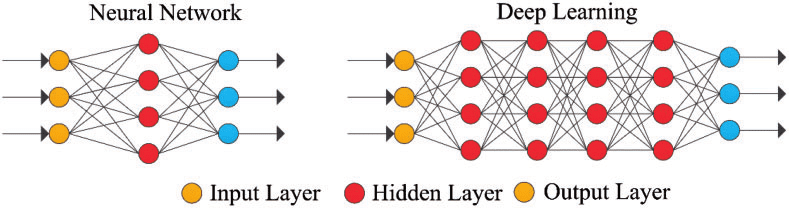
The outermost layers in yellow are the input or output layers, and the intermediate or hidden layers are in red. Deep Learning is responsible for the recent advances in computing, speech recognition, language processing and auditory identification, based on the definition of artificial neural networks or computational systems that reproduce the Way the human brain acts.
Another aspect of Deep Learning is the extraction of resources, which uses an algorithm to automatically create relevant parameters of information for training, learning and understanding, a task of the artificial intelligence engineer.
Deep Learning is an evolution of neural networks. The interest in deep learning has grown gradually in the media and several researches in the area have been disseminated and its application has reached cars, in the diagnosis of cancer and autism, among other applications[23]
The first deep learning algorithms with multiple layers of nonlinear assignments present their origins in Alexey Grigoryevich Ivakhnenko, who developed the data manipulation group method and Valentin Grigor ‘ Evich Lapa, author of the work Cybernetics and Forecasting Techniques in the year 1965.[24]
Both used thin and deep models with polynomial activation functions, which were investigated using statistical methods. Through these methods they selected in each layer the best resources and transmitted to the next layer, without using Backpropagation to “train” the complete network, but used minimum squares on each layer, where the previous ones were installed from Independently in the later layers, manually.
Figure 5: Structure of the first deep network known as Alexey Grigorevich Ivakhnenko
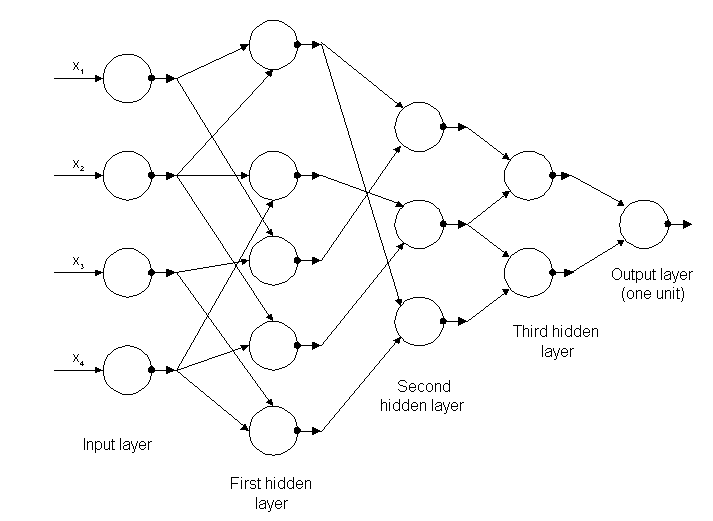
At the end of the decade of 1970 the winter of artificial intelligence occurred, a drastic reduction in funding for research on the subject. The impact has limited the advances in deep neural networks and Artificial intelligence.
The first convolutional neural networks were used by Kunihiko Fukushima, with several layers of grouping and convolutions, in 1979. He created an artificial neural network, called Neocognitron, with a hierarchical and multilayer layout, which enabled the computer to identify visual patterns. The networks were similar to modern versions, with “training” focused on the strategy of strengthening periodic activation in countless layers. Furthermore, the Fukushima design made it possible for the most relevant resources to be adapted manually by increasing the importance of certain connection[25]s.
Many Neocognitron guidelines are still in use, as top-down connections and new learning practices have promoted the realization of various neural networks. When several patterns are presented at the same time, the selective care model can separate them and identify the individual patterns, paying attention to each one. A more up-to-date Neocognitron can identify patterns with lack of data and complete the image by inserting the missing information, which is called inference.
The Backpropagation used for Deep Learning failure training progressed from 1970 onwards, when Seppo Linnainmaa wrote a thesis, inserting a FORTRAN code for Backpropagation, without succeeding until 1985. Rumelhart, Williams and Hinton then demonstrated Backpropagation in a neural network with representations of distribution.
This discovery allowed the debate on AI to reach cognitive psychology that initiated questions about human comprehension and its relationship with symbolic logic, as well as connections. In 1989, Yann LeCun performed a practical demonstration of Backpropagation, with the combination of convolutional neural networks to identify the written digits.
In this period there was again a shortage of funding for research in this area, known as the second winter of the IA, which occurred between 1985 and 1990, also affecting research in neural networks and Deep Learning. The expectations presented by some researchers did not reach the expected level, which deeply irritated investors.
In 1995, Dana Cortes and Vladimir Vapnik created the support Vector mac[26]hine or supporting vector machine that was a system for mapping and identifying similar information. The Long Short Term Memory-LSTM for periodic neural networks was elaborated in 1997, by Sepp Hochreiter and Juergen Schmidhube[27]r.
The next step in the evolution of Deep Learning occurred in 1999, when data processing and graphics processing units (GPUs) became faster. The use of GPUs and its rapid processing represented an increase in the speed of computers. Neural networks competed with support vector machines. The neural network was slower than a support vector machine, but they obtained better results and continued to evolve as more training information was added.
In the year 2000, a problem called Vanishing Gradient was identified. The assignments learned in lower layers were not transmitted to the upper layers However, it only occurred in those with gradient-based learning methods. The source of the problem was in some activation functions that reduced its input affecting the output range, generating large areas of input mapped in a very small range, causing a falling gradient. The solutions implemented to solve the issue were the pre-workout layer by layer and the development of a long and short term memory[28].
In 2009, Fei-Fei Li released ImageNet[29] with a free database of more than 14 million images, focused on the “training” of neural networks, pointing out how Big data would affect the operation of Machine Learning.
The velocity of the GPUs, up to the year 2011, continued to increase allowing the composition of convolutional neural networks without the need of pre-workout layer by layer. Thus, it became notorious that Deep Learning was advantageous in terms of efficiency and speed.
In the present day, Big Data processing and the progression of artificial intelligence are dependent on Deep Learning, which can elaborate intelligent systems and promote the creation of a fully autonomous artificial intelligence, which will create an impact on The whole society.
FLEXIVILITY OF NEURAL NETWORKS AND THEIR APPLICATIONS
Despite the existence of several classical techniques, the DEEP Learning structure and its basic unit, the neuron is generic and very flexible. By making a comparison with the human neuron that provides synapses we can identify some correlations between them.
Figure 6: Correlation between a human neuron and an artificial neural network
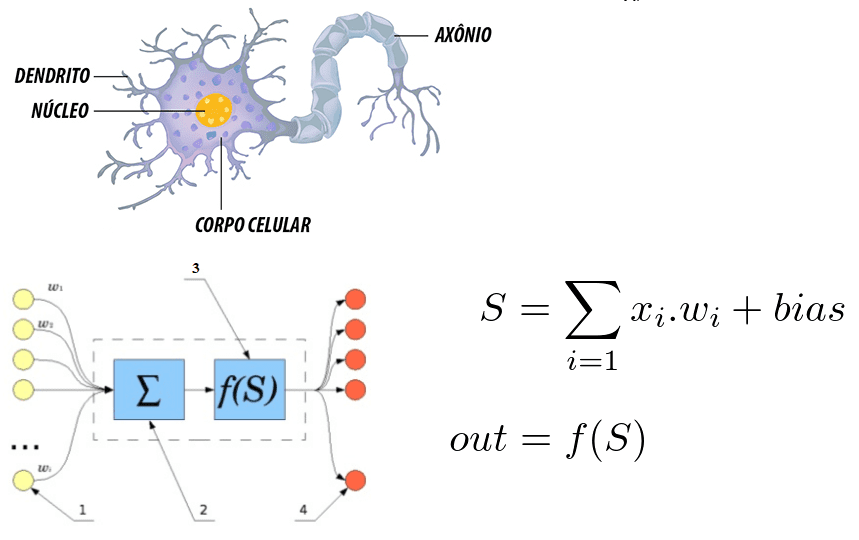
It is noted that the neuron is formed by the dendrites that are the points of entry, a nucleus that represents in the artificial neural networks the processing nucleus and the exit point that represented by the axon. In both systems the information enters, is processed and changes out.
Considering it as a mathematical equation, the neuron reflects the sum of the inputs multiplied by weights, and this value goes through an activation function. This sum was performed by McCulloch and Pitts in 1943[30]
In relation to the notorious interest on Deep Learning nowadays, Santana c[31]onsiders that it is due to two factors which are the amount of information available and the limitation of older techniques besides the current computational power to train networks Complex. The flexibility to interconnect multiple neurons in a more complex network is the differential of DEEP Learning structures. A convolutional neural network is widely used for facial recognition, image detection and assignment extraction.
A conventional neural network consists of several layers, called Layers. Depending on the issue to be solved the amount of layers may vary, being able to have up to hundreds of layers, being factors that influence in quantity the complexity of the problem, time and computational power
There are several different structures with countless purposes and their functioning also depends on the structure and all are based on neural networks.
Figure 7: Examples of neural networks
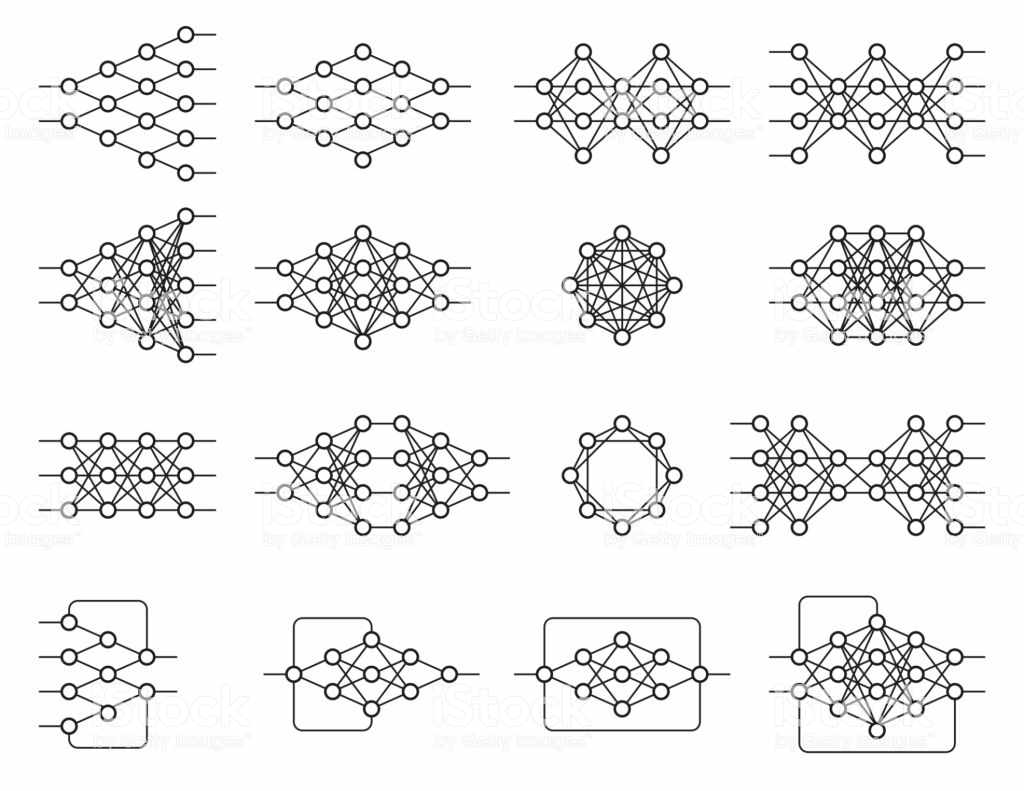
This architectural flexibility allows deep learning to solve various issues. Deep Learning is a general objective technique, but the most advanced scopes were: computer vision, speech recognition, natural language processing, recommendation systems.
The computational vision encompasses object recognition, semantic segmentation, especially autonomous cars. It can be affirmed that computational vision is part of artificial intelligence and is defined as a set of knowledge that seeks artificial modeling of human vision with the objective of imitating its functions, through the development of software and hardware Advanced[32].
Among the applications of the computational vision are the military use, marketing market, security, public services and in the production process. Autonomous vehicles represent the future of safer traffic, but it is still in the testing phase, as it encompasses several technologies applied to a function. The computational vision in these vehicles, since it allows the recognition of the path and the obstacles, improving the routes.
In the context of security, facial recognition systems have been increasingly highlighted, given the level of security in public and private places, also implemented in mobile devices. Similarly they can serve as a key to access financial transactions, while on social networks, it detects the presence of the user or his friends in photos.
In relation to the marketing market, a research developed by Image Intelligence pointed out that 3 billion images are shared daily by social networks and 80% contain indications that refer to specific companies, but without textual references. Specialized marketing companies offer real-time presence monitoring and management service. With computer vision technology, accuracy in image identification reaches 99%.
In public services its use covers the security of the site by monitoring cameras, vehicle traffic through stereoscopic images that make the vision system efficient.
In the production process, companies from different branches employ the computational vision as a quality control instrument. In any branch, the most advanced software associated with the ever-increasing processing capacity of the hardware increases the use of computational vision.
Monitoring systems allow the recognition of pre-established standards, and point out failures that would not be identifiable when looking at an employee in the production line. In the same context, applied to inventory control is the replacement automation project. A real-time inventory and sales control enables the technology to control the operations of a given company, thus increasing its profits. There are other applications in the field of medicine, education and e-commerce.
Conclusions
The present study sought to elucidate what Deep Learning is and to point out its applications in the current world. Deep learning techniques continue to progress in particular with the use of multiple layers. However, there are still limitations in the use of deep neural networks, given that they are only one way to learn several changes to be implemented in the input vector. Changes provided by a range of parameters that are updated in the training period.
It is undeniable that artificial intelligence is a closer reality, but it lacks a long way to go. The acceptance of deep learning in various fields of knowledge allows society, as a whole, to benefit from the wonders of modern technology.
In relation to artificial intelligence, it is verified that this technology capable of learning, although very important has a linear and non-moldable nature as of human beings, which represents a great differential and essential for some areas of knowledge, the That cannot yet be implemented in deep learning.
In any way, the use of the deep learning methods will enable the machines to assist society in various activities as demonstrated, expanding the cognitive capacity of man and an even greater development in these areas of knowledge.
BIBLIOGRAPHICAL REFERENCES
ALIGER. Saiba o que é visão computacional e como ela pode ser usada. 2018. Disponível em: https://www.aliger.com.br/blog/saiba-o-que-e-visao-computacional/
BITTENCOURT, Guilherme. Breve história da Inteligência Artificial. 2001
CARVALHO, André Ponce de L. Redes Neurais Artificiais. Disponível em: http://conteudo.icmc.usp.br/pessoas/andre/research/neural/
CHUNG, J; GULCEHE, C; CHO, K; BENGIO, Y. Gated feedback recurrent neural networks. In Proceedings of the 32nd International Conference on International Conference on Machine Learning, volume 37 of ICML, pages 2067–2075. 2015
CULTURA ANALITICA. Entenda o que é deep learning e como funciona. Disponível em: https://culturaanalitica.com.br/deep-learning-oquee-como-funciona/cultura-analitica-redes-neurais-simples-profundas/
DENG, Jia et al. Imagenet: Um banco de dados de imagens hierárquicas em grande escala. In: 2009 Conferência IEEE sobre visão computacional e reconhecimento de padrões . Ieee,. p. 248-255. 2009
DETTMERS, Tim. Aprendizagem profunda em poucas palavras: Historia e Treinamento. Disponível em: https://devblogs.nvidia.com/deep-learning-nutshell-history-training/
FUKUSHIMA, Kunihiko. Neocognitron: Um modelo de rede neural auto-organizada para um mecanismo de reconhecimento de padrões não afetado pela mudança de posição. Cibernética biológica , v. 36, n. 4, p. 193-202, 1980.
GARTNER. Gartner diz que a AI Technologies estará em quase todos os novos produtos de software até 2020. Disponível em: https://www.gartner.com/en/newsroom/press-releases/2017-07-18-gartner-says-ai-technologies-will-be-in-almost-every-new-software-product-by-2020
GOODFELLOW, I; BENGIOo, Y; COURVILLE, A. Deep Learning. MIT Press. Disponível em: http://www.deeplearningbook.org
GRAVES, A. Sequence transduction with recurrent neural networks. arXiv preprint arXiv:1211.3711, 2012.
HOCHREITER, Sepp. O problema do gradiente de fuga durante a aprendizagem de redes neurais recorrentes e soluções de problemas. Revista Internacional de Incerteza, Fuzziness and Knowledge-Based Systems , v. 6, n. 02, p. 107-116, 1998.
HEBB, Donald O. The organization of behavior; a neuropsycholocigal theory. A Wiley Book in Clinical Psychology., p. 62-78, 1949.
HINTON, Geoffrey E; SALAKHUTDINOV, Ruslan R. Reduzindo a dimensionalidade dos dados com redes neurais. ciência , v. 313, n. 5786, p. 504-507, 2006.
HOCHREITER, Sepp; SCHMIDHUBER, Jürgen. Longa memória de curto prazo. Computação Neural, v. 9, n. 8, p. 1735-1780, 1997.
INTRODUCTION to artificial neural networks, Proceedings Electronic Technology Directions to the Year 2000, Adelaide, SA, Australia, 1995, pp. 36-62. Disponível em: https://ieeexplore.ieee.org/document/403491
KOROLEV, Dmitrii. Set of different neural nets. Neuron network. Deep learning. Cognitive technology concept. Vector illustration Disponível em: https://stock.adobe.com/br/images/set-of-different-neural-nets-neuron-network-deep-learning-cognitive-technology-concept-vector-illustration/145011568
LIU, C.; CAO, Y; LUO Y; CHEN, G; VOKKARANE, V, MA, Y; CHEN, S; HOU, P. A new Deep Learning-based food recognition system for dietary assessment on an edge computing service infrastructure. IEEE Transactions on Services Computing, PP(99):1–13. 2017
LORENA, Ana Carolina; DE CARVALHO, André CPLF. Uma introdução às support vector machines. Revista de Informática Teórica e Aplicada, v. 14, n. 2, p. 43-67, 2007.
MARVIN, Minsky; SEYMOUR, Papert. Perceptrons. 1969. Disponível em: http://134.208.26.59/math/AI/AI.pdf
MCCULLOCH, Warren S; PITTS, Walter. Um cálculo lógico das idéias imanentes na atividade nervosa. O boletim de biofísica matemática, v. 5, n. 4, p. 115-133, 1943.
NIELSEN, Michael. How the backpropagation algorithm works. 2018. Disponível em: http://neuralnetworksanddeeplearning.com/chap2.html
NIPS. 2009. Disponível em: http://media.nips.cc/Conferences/2009/NIPS-2009-Workshop-Book.pdf
PANG, Y; SUN, M; JIANG, X; LI, X.. Convolution in convolution for network in network. IEEE Transactions on Neural Networks and Learning Systems, PP(99):1–11. 2017
SANTANA, Marlesson. Deep Learning: do Conceito às Aplicações. 2018. Disponível em: https://medium.com/data-hackers/deep-learning-do-conceito-%C3%A0s-aplica%C3%A7%C3%B5es-e8e91a7c7eaf
UDACITY. Conheça 8 aplicações de Deep Learning no mercado. Disponível em: https://br.udacity.com/blog/post/aplicacoes-deep-learning-mercado
YANG, S; LUO, P; LOY, C.C; TANG, X. From facial parts responses to face detection: A Deep Learning approach. In Proceedings of 2015 IEEE International Conference on Computer Vision (ICCV), pages 3676–3684. 2015
- Liu, C., Cao, Y., Luo, Y., Chen, G., Vokkarane, V., Ma, Y., Chen, S., and Hou, P. (2017). A new Deep Learning-based food recognition system for dietary assessment on an edge computing service infrastructure. IEEE Transactions on Services Computing, PP(99):1–13.
- Yang, S., Luo; P., Loy, C.-C; Tang, X. From facial parts responses to face detection: A Deep Learning approach. In Proceedings of 2015 IEEE International Conference on Computer Vision (ICCV), p. 3676
- Chung, J; Gulcehre, C; Cho, K; Bengio, Y. Gated feedback recurrent neural networks. In Proceedings of the 32nd International Conference on International Conference on Machine Learning, volume 37 of ICML, p. 2069
- PANG, Y; Sun, M., Jiang, X., and Li, X. (2017). Convolution in convolution for network in network. IEEE Transactions on Neural Networks and Learning Systems, PP(99):5. 2017 ↑
- Goodfellow, I., Bengio, Y., and Courville, A. Deep Learning. MIT Press. http://www.deeplearningbook.org.
- GRAVES, A. Sequence transduction with recurrent neural networks. arXiv preprint arXiv:1211.3711, 2012.
- GARTNER. Gartner diz que a AI Technologies estará em quase todos os novos produtos de software até 2020. Disponível em: https://www.gartner.com/en/newsroom/press-releases/2017-07-18-gartner-says-ai-technologies-will-be-in-almost-every-new-software-product-by-2020
- MCCULLOCH, Warren S; PITTS, Walter. Um cálculo lógico das idéias imanentes na atividade nervosa. O boletim de biofísica matemática , v. 5, n. 4, p. 115-133, 1943.
- HEBB, Donald O. The organization of behavior; a neuropsycholocigal theory. A Wiley Book in Clinical Psychology., p. 62-78, 1949.
- BITTENCOURT, Guilherme. Breve história da Inteligência Artificial. 2001.p. 24
- Idbiem p. 25
- MARVIN, Minsky; SEYMOUR, Papert. Perceptrons. 1969. Disponível em: http://134.208.26.59/math/AI/AI.pdf
- Introduction to artificial neural networks,” Proceedings Electronic Technology Directions to the Year 2000, Adelaide, SA, Australia, 1995, pp. 36-62. Disponível em: https://ieeexplore.ieee.org/document/403491
- BITTENCOURT, Guilherme op cit. p. 32
- NIELSEN, Michael. How the backpropagation algorithm works. 2018. Disponível em: http://neuralnetworksanddeeplearning.com/chap2.html
- HINTON, Geoffrey E; SALAKHUTDINOV, Ruslan R. Reduzindo a dimensionalidade dos dados com redes neurais. ciência , v. 313, n. 5786, p. 504-507, 2006.
- NIPS. 2009. Disponível em: http://media.nips.cc/Conferences/2009/NIPS-2009-Workshop-Book.pdf
- Santana, Marlesson. Deep Learning: do Conceito às Aplicações. 2018. Disponivel em: https://medium.com/data-hackers/deep-learning-do-conceito-%C3%A0s-aplica%C3%A7%C3%B5es-e8e91a7c7eaf
- SANTANA, Marlesson. Deep Learning: do Conceito às Aplicações. 2018. Disponível em: https://medium.com/data-hackers/deep-learning-do-conceito-%C3%A0s-aplica%C3%A7%C3%B5es-e8e91a7c7eaf
- idem
- UDACITY. Conheça 8 aplicações de Deep Learning no mercado. Disponível em: https://br.udacity.com/blog/post/aplicacoes-deep-learning-mercado
- Dettmers, Tim. Aprendizagem profunda em poucas palavras: Historia e Treinamento. Disponível em: https://devblogs.nvidia.com/deep-learning-nutshell-history-training/
- FUKUSHIMA, Kunihiko. Neocognitron: Um modelo de rede neural auto-organizada para um mecanismo de reconhecimento de padrões não afetado pela mudança de posição. Cibernética biológica , v. 36, n. 4, p. 193-202, 1980.
- LORENA, Ana Carolina; DE CARVALHO, André CPLF. Uma introdução às support vector machines. Revista de Informática Teórica e Aplicada, v. 14, n. 2, p. 43-67, 2007.
- HOCHREITER, Sepp; SCHMIDHUBER, Jürgen. Longa memória de curto prazo. Computação Neural, v. 9, n. 8, p. 1735-1780, 1997.
- HOCHREITER, Sepp. O problema do gradiente de fuga durante a aprendizagem de redes neurais recorrentes e soluções de problemas. Revista Internacional de Incerteza, Fuzziness and Knowledge-Based Systems , v. 6, n. 02, p. 107-116, 1998.
- DENG, Jia et al. Imagenet: Um banco de dados de imagens hierárquicas em grande escala. In: 2009 Conferência IEEE sobre visão computacional e reconhecimento de padrões . Ieee, 2009. p. 248-255.
- MCCULLOCH, Warren S.; PITTS, Walter. Um cálculo lógico das idéias imanentes na atividade nervosa. O boletim de biofísica matemática , v. 5, n. 4, p. 115-133, 1943.
- SANTANA, Marlesson. Deep Learning: do Conceito às Aplicações. 2018. Disponivel em: https://medium.com/data-hackers/deep-learning-do-conceito-%C3%A0s-aplica%C3%A7%C3%B5es-e8e91a7c7eaf
- ALIGER. Saiba o que é visão computacional e como ela pode ser usada. 2018. Disponível em: https://www.aliger.com.br/blog/saiba-o-que-e-visao-computacional/
[1] Bachelor of Business Administration.
Submitted: May, 2019
Approved: May, 2019